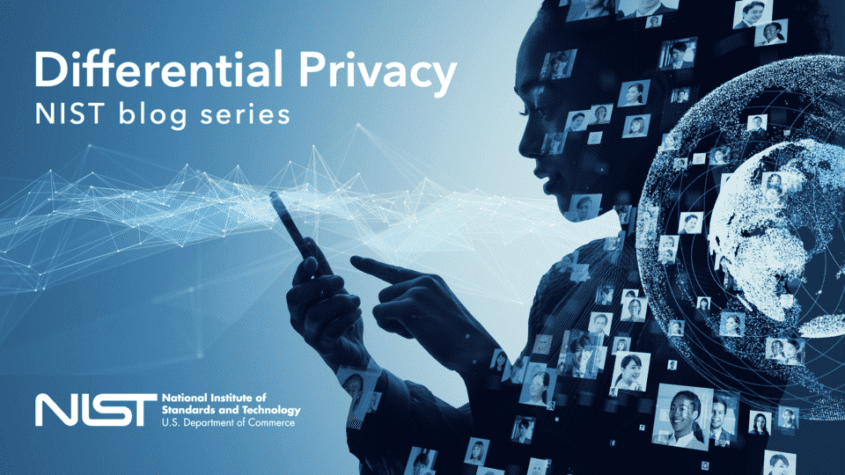
Does your organization want to aggregate and analyze data to learn trends, but in a way that protects privacy? Or perhaps you are already using differential privacy tools, but want to expand (or share) your knowledge? In either case, this blog series is for you.
Why are we doing this series? Last year, NIST launched a Privacy Engineering Collaboration Space to aggregate open source tools, solutions, and processes that support privacy engineering and risk management. As moderators for the Collaboration Space, we’ve helped NIST gather differential privacy tools under the topic area of de-identification. NIST also has published the Privacy Framework: A Tool for Improving Privacy through Enterprise Risk Management and a companion roadmap that recognized a number of challenge areas for privacy, including the topic of de-identification. Now we’d like to leverage the Collaboration Space to help close the roadmap’s gap on de-identification. Our end-game is to support NIST in turning this series into more in-depth guidance on differential privacy.
Each post will begin with conceptual basics and practical use cases, aimed at helping professionals such as business process owners or privacy program personnel learn just enough to be dangerous (just kidding). After covering the basics, we’ll look at available tools and their technical approaches for privacy engineers or IT professionals interested in implementation details. To get everyone up to speed, this first post will provide background on differential privacy and describe some key concepts that we’ll use in the rest of the series.
The Challenge
How can we use data to learn about a population, without learning about specific individuals within the population? Consider these two questions:
- “How many people live in Vermont?”
- “How many people named Joe Near live in Vermont?”
The first reveals a property of the whole population, while the second reveals information about one person. We need to be able to learn about trends in the population while preventing the ability to learn anything new about a particular individual. This is the goal of many statistical analyses of data, such as the statistics published by the U.S. Census Bureau, and machine learning more broadly. In each of these settings, models are intended to reveal trends in populations, not reflect information about any single individual.
But how can we answer the first question “How many people live in Vermont?” — which we’ll refer to as a query — while preventing the second question from being answered “How many people name Joe Near live in Vermont?” The most widely used solution is called de-identification (or anonymization), which removes identifying information from the dataset. (We’ll generally assume a dataset contains information collected from many individuals.) Another option is to allow only aggregate queries, such as an average over the data. Unfortunately, we now understand that neither approach actually provides strong privacy protection. De-identified datasets are subject to database-linkage attacks. Aggregation only protects privacy if the groups being aggregated are sufficiently large, and even then, privacy attacks are still possible [1, 2, 3, 4].
Differential Privacy
Differential privacy [5, 6] is a mathematical definition of what it means to have privacy. It is not a specific process like de-identification, but a property that a process can have. For example, it is possible to prove that a specific algorithm “satisfies” differential privacy.
Informally, differential privacy guarantees the following for each individual who contributes data for analysis: the output of a differentially private analysis will be roughly the same, whether or not you contribute your data. A differentially private analysis is often called a mechanism, and we denote it ℳ.
Figure 1 illustrates this principle. Answer “A” is computed without Joe’s data, while answer “B” is computed with Joe’s data. Differential privacy says that the two answers should be indistinguishable. This implies that whoever sees the output won’t be able to tell whether or not Joe’s data was used, or what Joe’s data contained.
We control the strength of the privacy guarantee by…
Privacy 2024 Recap – some significant decisions, slow progress for reform
The past year saw a few court decisions of note as well as halting progress toward privacy…