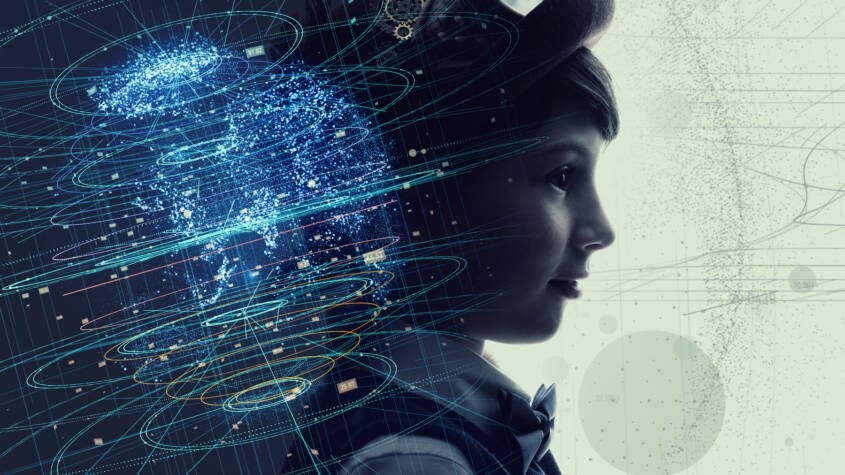
At a glance
- Anyone involved in the decision-making pipeline has a role to play in contributing to an explanation of a decision supported by an AI model’s result.
- This includes what we have called the AI development team, as well as those responsible for how decision-making is governed in your organisation.
- We recognise that every organisation has different structures for their AI development and governance teams, and in smaller organisations several of the functions we outline will be covered by one person.
- Many organisations will outsource the development of their AI system. In this case, you as the data controller have the primary responsibility for ensuring that the AI system you use is capable of producing an explanation for the decision recipient.
Checklist
☐ We have identified the people who are in key roles across the decision-making pipeline and how they are responsible for contributing to an explanation of the AI system.
☐ We have ensured that different people along the decision-making pipeline are able to carry out their role in producing and delivering explanations, particularly those in AI development teams, those giving explanations to decision recipients, and our DPO and compliance teams.
☐ If we are buying the AI system from a third party, we know we have the primarily responsibility for ensuring that the AI system is capable of producing explanations.
In more detail
- Who should participate in explanation extraction and delivery?
- What if we use an AI system supplied by a third party?
Who should participate in explanation extraction and delivery?
People involved in every part of the decision-making pipeline, including the AI model’s design and implementation processes, have a role to play in providing explanations to individuals who receive a decision supported by an AI model’s result.
In this section, we will describe the various roles in the end-to-end process of providing an explanation. In some cases, part of this process may not sit within your organisation, for example if you have procured the system from an external vendor. More information on this process is provided later in Part 3.
The roles discussed range from those involved in the initial decision to use an AI system to solve a problem and the teams building the system, to those using the output of the system to inform the final decision and those who govern how decision-making is done in your organisation. Depending on your organisation, the roles outlined below might be configured in different ways, or concentrated in just one or two people.
Please note that this is not an exhaustive list of all individuals that may be involved in contributing to an explanation for a decision made by an AI system. There may be other roles unique to your organisation or sector that are not outlined here. The roles listed below are the main ones we feel every organisation should consider when implementing an AI system to make decisions about individuals.
Overview of the roles involved in providing an explanation
Product manager: defines the product requirements for the AI system and determines how it should be managed, including the explanation requirements and potential impacts of the system’s use on affected individuals. The product manager is also be responsible throughout the AI system’s lifecycle. They are responsible for ensuring it is properly maintained, and that improvements are made where relevant. They also need to ensure that the system is procured and retired in compliance with all relevant legislation, including GDPR and DPA 2018.
AI development team: The AI development team performs several functions, including:
- collecting, procuring and analysing the data that you input into your AI system, which must be representative, reliable, relevant, and up-to-date;
- bringing in domain expertise to ensure the AI system is capable of delivering the types of explanations required. Domain experts could, for example, be doctors, lawyers, economists or engineers;
- building and maintaining the data architecture and infrastructure that ensure the system performs as intended and that explanations can be extracted;
- building, training and optimising the models you deploy in your AI system, prioritising interpretable methods;
- testing the model, deploying it, and extracting explanations from it; and
- supporting implementers in deploying the AI system in practice.
Please note that the AI development team may sit within your organisation, or be part of another organisation if you purchased the system from a third party. If you procure a system from a third party, you still need to ensure that you understand how the system works and how you can extract the meaningful information necessary to provide an appropriate explanation.
Implementer: where there is a human in the loop (ie the decision is not fully automated) the implementer relies on the model developed to supplement or complete a task in their everyday work life. In order to extract an explanation, implementers either directly use the model, if it is inherently interpretable and simple, or use supplementary tools and methods that enable explanation, if it is not. The tools and methods provide implementers with information that represents components of the rationale behind the model’s results, such as relative feature importance. Implementers take this information and consider it together with other evidence to make a decision on how to proceed.
Where a system is developed by a third party vendor, you should ensure that they provide sufficient training and support so that your implementers are able to understand the model you are using. If you do not have this support in place, your implementers may not have the skills and knowledge to deploy the system responsibly and to provide accurate and context sensitive explanations to your decision recipients.
Compliance teams, including DPO:..
Taming the AI Beast: A Risk-Based Guide to Smarter AI Governance
In today’s digital age, Artificial Intelligence (AI) is revolutionizing industries, …